Novel AI architecture locates anatomically important points in the skull, face, and jaw bones accurately, helping doctors plan orthodontic treatments
SPIE--International Society for Optics and Photonics 3d Human Skeleton Model
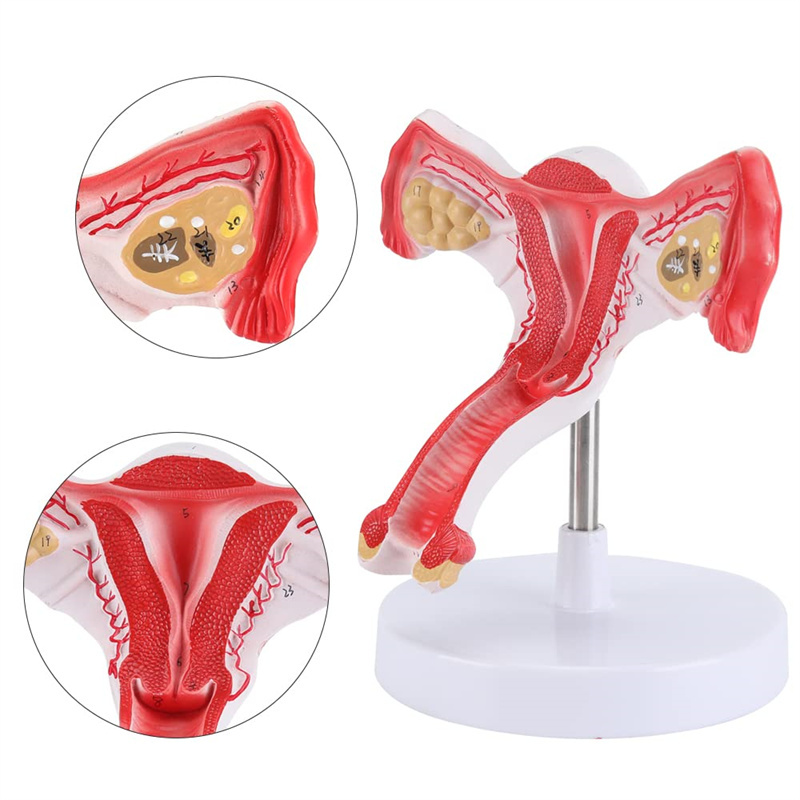
image: A novel deep learning architecture, the “relational reasoning network,” can learn the spatial relationships between key anatomical landmarks of craniomaxillofacial bones from computed tomography images. This enables a trained model to find these landmarks automatically and accurately in images from all types of patients, even those with deformities that would trip up other deep learning models. view more
Credit: Credit: Torosdagli et al., doi 10.1117/1.JMI.10.2.024002.
Millions of people around the world undergo some type of orthodontic treatment each year due largely to developmental deformities in the jaw, skull, or face. Computed tomography (CT) imaging is the go-to technique for surgeons when planning such treatments, especially surgeries. This is because CT provides 3D images of the bones and teeth, which helps the surgeon analyze complex cases in detail and determine the best treatment procedure based on that.
During the CT scan, surgeons typically try to pinpoint specific anatomical landmarks in the images. These are distinct points in the human body that can be used as a reference to make measurements and assess a condition or deformity. However, finding these landmarks can be time-consuming and requires considerable skill. Many researchers have therefore attempted to automate this process with artificial intelligence (AI), achieving varying levels of success.
A common problem with existing AI approaches is that they rely on a process known as “segmentation.” In medical image analysis, segmentation implies separating an image into different relevant regions, such as individual bones or specific tissue groups. While this approach works well enough for most people, they tend to fail for patients with implants or deformities, including missing or broken bones. But what if we could find a way to perform anatomical landmarking without needing to segment the image first?
This was the goal of a study conducted by a research team from Northwestern University in the USA, as reported in Journal of Medical Imaging (JMI). The researchers hypothesized that a deep learning AI model should be able to learn the spatial relationships among the anatomical landmarks of the craniomaxillofacial (CMF) bones (bones of the skull, face, and jaw) without requiring an explicit image segmentation. “This approach where an AI model can automatically learn the relationships between anatomies and their underlying reasons is known as ‘relational reasoning.’ While well-known in robotics, relational reasoning has hardly been considered in medical imaging,” explains corresponding author Ulas Bagci, Associate Professor at Northwestern University's Radiology and Biomedical Engineering Department.
When designing the model, the researchers sought to answer the following questions: (a) Is it possible to identify all anatomical landmarks based on learning only a few of them? (b) Which landmarks are the most informative for the model? An important aspect of their strategy was to implement a model architecture that could learn both “local” and “global” relations. A local relation refers to the relative position between a pair of landmarks, whereas a global relation refers to the position of a landmark in relation to those of all other landmarks.
Accordingly, the architecture of the proposed AI model, which they called a “relational reasoning network” (RRN), has two stages incorporating core blocks known as “relational units.” In the first stage, the model learns about local relations between the members of a given set of landmarks. In the second stage, the model learns about global relations between each landmark and the rest. The team trained the model with a large dataset of landmarks derived from an artificially augmented 250-image dataset of CT scans. A good portion of the patients included in the dataset presented with birth defects, developmental deformities, missing bones or teeth, and previous surgical interventions.
The researchers tested several combinations of landmarks to determine the combination with best performance. They also compared the model’s performance to conventional AI-based landmarking methods. Overall, the accuracy of the RRN was remarkable, in line with or better than that of previously reported techniques. Moreover, the model showed good generalizability, meaning that it performed well when tested with previously unseen data gathered in different conditions. The researchers suggest that this was because the RRN framework could learn the functional relationships between CMF landmarks that are still present to some degree in cases of large deformities.
“With an error less than 2 mm per anatomical landmark in the most difficult cases, the method developed in our study could not only help surgeons save time but also avoid incorrect landmarking that might arise from segmentation failures. Moreover, it sets a precedent for future AI models aimed at learning relationships between anatomical landmarks in other parts of the body,” concludes an optimistic Bagci.
Let us hope more such studies will help surgeons increasingly leverage the power of AI in medical image analysis.
Read the Gold Open Access article by N. Torsdagli et al., “Relational reasoning network for anatomical landmarking,” J. Med. Imaging 10(2) 024002 (2023), doi 10.1117/1.JMI.10.2.024002.
Relational reasoning network for anatomical landmarking
Disclaimer: AAAS and EurekAlert! are not responsible for the accuracy of news releases posted to EurekAlert! by contributing institutions or for the use of any information through the EurekAlert system.
Daneet Steffens SPIE--International Society for Optics and Photonics daneets@spie.org Office: 360-685-5478
SPIE--International Society for Optics and Photonics
Copyright © 2023 by the American Association for the Advancement of Science (AAAS)
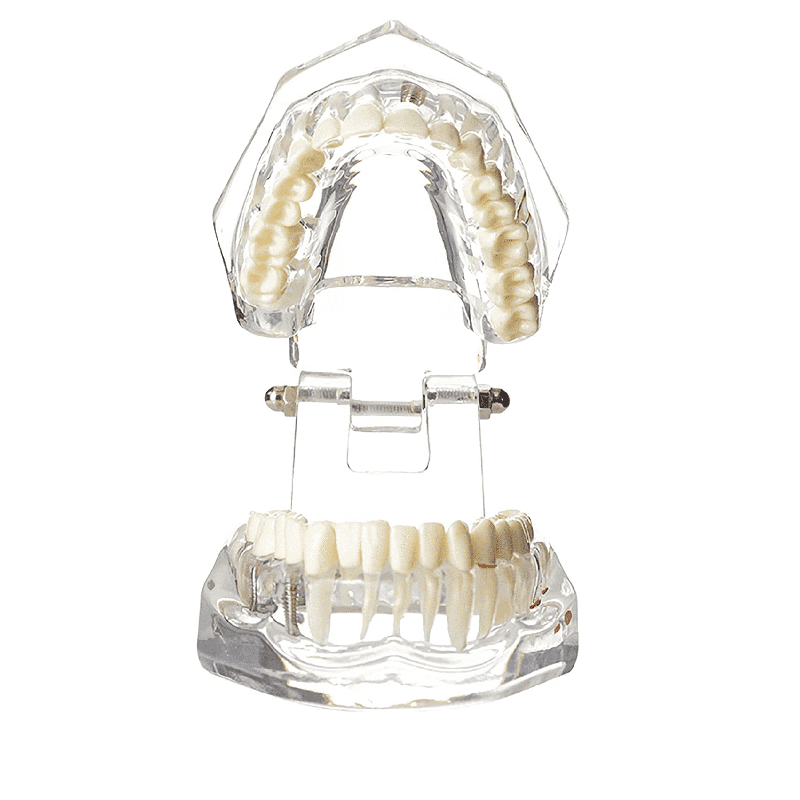
Suture Training Kit Copyright © 2023 by the American Association for the Advancement of Science (AAAS)